All Episodes
BI 119 Henry Yin: The Crisis in Neuroscience
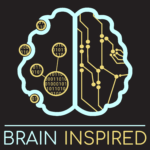
Henry and I discuss why he thinks neuroscience is in a crisis (in the Thomas Kuhn sense of scientific paradigms, crises, and revolutions). Henry thinks our current concept of the brain as an input-output device, with cognition in the middle, is mistaken. He points to the failure of neuroscience to successfully explain behavior despite decades of research. Instead, Henry proposes the brain is one big hierarchical set of control loops, trying to control their output with respect to internally generated reference signals. He was inspired by control theory, but points out that most control theory for biology is flawed by not recognizing that the reference signals are internally generated. Instead, most control theory approaches, and neuroscience research in general, assume the reference signals are what gets externally supplied… by the experimenter.
BI 118 Johannes Jäger: Beyond Networks
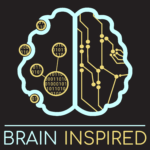
Johannes (Yogi) is a freelance philosopher, researcher & educator. We discuss many of the topics in his online course, Beyond Networks: The Evolution of Living Systems. The course is focused on the role of agency in evolution, but it covers a vast range of topics: process vs. substance metaphysics, causality, mechanistic dynamic explanation, teleology, the important role of development mediating genotypes, phenotypes, and evolution, what makes biological organisms unique, the history of evolutionary theory, scientific perspectivism, and a view toward the necessity of including agency in evolutionary theory. I highly recommend taking his course. We also discuss the role of agency in artificial intelligence, how neuroscience and evolutionary theory are undergoing parallel re-evaluations, and Yogi answers a guest question from Kevin Mitchell.
BI 117 Anil Seth: Being You
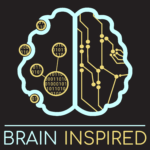
Anil and I discuss a range of topics from his book, BEING YOU A New Science of Consciousness. Anil lays out his framework for explaining consciousness, which is embedded in what he calls the “real problem” of consciousness. You know the “hard problem”, which was David Chalmers term for our eternal difficulties to explain why we have subjective awareness at all instead of being unfeeling, unexperiencing machine-like organisms. Anil’s “real problem” aims to explain, predict, and control the phenomenal properties of consciousness, and his hope is that, by doing so, the hard problem of consciousness will dissolve much like the mystery of explaining life dissolved with lots of good science.
BI 116 Michael W. Cole: Empirical Neural Networks
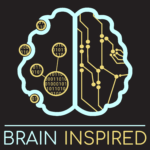
Mike and I discuss his modeling approach to study cognition. Many people I have on the podcast use deep neural networks to study brains, where the idea is to train or optimize the model to perform a task, then compare the model properties with brain properties. Mike’s approach is different in at least two ways. For one, he builds the architecture of his models using structural connectivity data from fMRI recordings. Two, he doesn’t train his models; instead, he uses functional connectivity data from the fMRI recordings to assign weights between nodes of the network (in deep learning, the weights are learned through lots of training). Mike calls his networks empirically-estimated neural networks (ENNs), and/or network coding models. We walk through his approach, what we can learn from models like ENNs, discuss some of his earlier work on cognitive control and our ability to flexibly adapt to new task rules through instruction, and he fields questions from Kanaka Rajan, Kendrick Kay, and Patryk Laurent.
BI 115 Steve Grossberg: Conscious Mind, Resonant Brain
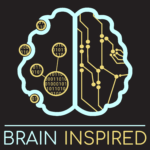
Steve and I discuss his book Conscious Mind, Resonant Brain: How Each Brain Makes a Mind. The book is a huge collection of his models and their predictions and explanations for a wide array of cognitive brain functions. Many of the models spring from his Adaptive Resonance Theory (ART) framework, which explains how networks of neurons deal with changing environments while maintaining self-organization and retaining learned knowledge. ART led Steve to the hypothesis that all conscious states are resonant states, which we discuss. There are also guest questions from György Buzsáki, Jay McClelland, and John Krakauer.
BI 114 Mark Sprevak and Mazviita Chirimuuta: Computation and the Mind
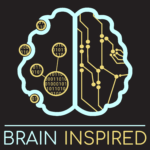
Mark and Mazviita discuss the philosophy and science of mind, and how to think about computations with respect to understanding minds. Current approaches to explaining brain function are dominated by computational models and the computer metaphor for brain and mind. But there are alternative ways to think about the relation between computations and brain function, which we explore in the discussion. We also talk about the role of philosophy broadly and with respect to mind sciences, pluralism and perspectival approaches to truth and understanding, the prospects and desirability of naturalizing representations (accounting for how brain representations relate to the natural world), and much more.
BI 113 David Barack and John Krakauer: Two Views On Cognition
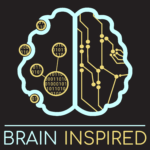
David and John discuss some of the concepts from their recent paper Two Views on the Cognitive Brain, in which they argue the recent population-based dynamical systems approach is a promising route to understanding brain activity underpinning higher cognition. We discuss mental representations, the kinds of dynamical objects being used for explanation, and much more, including David’s perspectives as a practicing neuroscientist and philosopher.
BI ViDA Panel Discussion: Deep RL and Dopamine
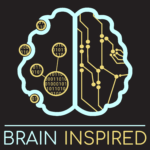
What can artificial intelligence teach us about how the brain uses dopamine to learn? Recent advances in artificial intelligence have yielded novel algorithms for reinforcement
learning (RL), which leverage the power of deep learning together with reward prediction error signals in order to achieve unprecedented performance in complex tasks. In the brain, reward prediction error signals are thought to be signaled by midbrain dopamine neurons and support learning. Can these new advances in Deep RL help us understand the role that dopamine plays in learning? In this panel experts in both theoretical and experimental dopamine research will
discuss this question.
BI 112 Ali Mohebi and Ben Engelhard: The Many Faces of Dopamine
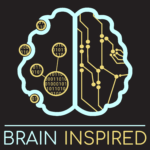
Ali and Ben discuss the ever-expanding discoveries about the roles dopamine plays for our cognition. Dopamine is known to play a role in learning – dopamine (DA) neurons fire when our reward expectations aren’t met, and that signal helps adjust our expectation. Roughly, DA corresponds to a reward prediction error. The reward prediction error has helped reinforcement learning in AI develop into a raging success, specially with deep reinforcement learning models trained to out-perform humans in games like chess and Go. But DA likely contributes a lot more to brain function. We discuss many of those possible roles, how to think about computation with respect to neuromodulators like DA, how different time and spatial scales interact, and more.
BI NMA 06: Advancing Neuro Deep Learning Panel
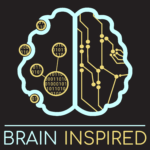
This is the 6th in a series of panel discussions in collaboration with Neuromatch Academy, the online computational neuroscience summer school. This is the 3rd of 3 in the deep learning series. In this episode, the panelists discuss their experiences with advanced topics in deep learning; unsupervised & self-supervised learning, reinforcement learning, continual learning/causality.
BI NMA 05: NLP and Generative Models Panel
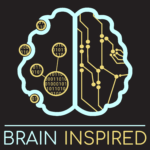
This is the 5th in a series of panel discussions in collaboration with Neuromatch Academy, the online computational neuroscience summer school. This is the 2nd of 3 in the deep learning series. In this episode, the panelists discuss their experiences “doing more with fewer parameters: Convnets, RNNs, attention & transformers, generative models (VAEs & GANs).
BI NMA 04: Deep Learning Basics Panel
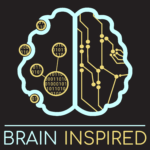
This is the 4th in a series of panel discussions in collaboration with Neuromatch Academy, the online computational neuroscience summer school. This is the first of 3 in the deep learning series. In this episode, the panelists discuss their experiences with some basics in deep learning, including Linear deep learning, Pytorch, multi-layer-perceptrons, optimization, & regularization.
BI 111 Kevin Mitchell and Erik Hoel: Agency, Emergence, Consciousness
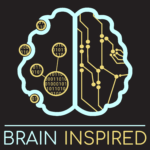
Erik, Kevin, and I discuss… well a lot of things.
We talk about both books, then dive deeper into topics like whether brains evolved for moving our bodies vs. consciousness, how information theory is lending insights to emergent phenomena, and the role of agency with respect to what counts as intelligence.
BI NMA 03: Stochastic Processes Panel
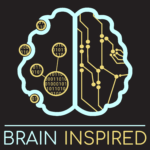
This is the third in a series of panel discussions in collaboration with Neuromatch Academy, the online computational neuroscience summer school. In this episode, the panelists discuss their experiences with stochastic processes, including Bayes, decision-making, optimal control, reinforcement learning, and causality.
BI NMA 02: Dynamical Systems Panel
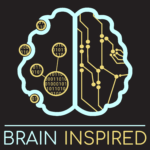
This is the second in a series of panel discussions in collaboration with Neuromatch Academy, the online computational neuroscience summer school. In this episode, the panelists discuss their experiences with linear systems, real neurons, and dynamic networks.
BI NMA 01: Machine Learning Panel
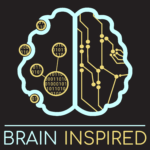
This is the first in a series of panel discussions in collaboration with Neuromatch Academy, the online computational neuroscience summer school. In this episode, the panelists discuss their experiences with model fitting, GLMs/machine learning, dimensionality reduction, and deep learning.
BI 110 Catherine Stinson and Jessica Thompson: Neuro-AI Explanation
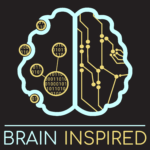
Catherine, Jess, and I use some of the ideas from their recent papers to discuss how different types of explanations in neuroscience and AI could be unified into explanations of intelligence, natural or artificial. Catherine has written about how models are related to the target system they are built to explain. She suggests both the model and the target system should be considered as instantiations of a specific kind of phenomenon, and explanation is a product of relating the model and the target system to that specific aspect they both share. Jess has suggested we shift our focus of explanation from objects – like a brain area or a deep learning model – to the shared class of phenomenon performed by those objects. Doing so may help bridge the gap between the different forms of explanation currently used in neuroscience and AI. We also discuss Henk de Regt’s conception of scientific understanding and its relation to explanation (they’re different!), and plenty more.
BI 109 Mark Bickhard: Interactivism
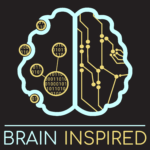
Mark and I discuss a wide range of topics surrounding his Interactivism framework for explaining cognition. Interactivism stems from Mark’s account of representations and how what we represent in our minds is related to the external world – a challenge that has plagued the mind-body problem since the beginning. Basically, representations are anticipated interactions with the world, that can be true (if enacting one helps an organism maintain its thermodynamic relation with the world) or false (if it doesn’t). And representations are functional, in that they function to maintain far from equilibrium thermodynamics for the organism for self-maintenance. Over the years, Mark has filled out Interactivism, starting with a process metaphysics foundation and building from there to account for representations, how our brains might implement representations, and why AI is hindered by our modern “encoding” version of representation. We also compare interactivism to other similar frameworks, like enactivism, predictive processing, and the free energy principle