All Episodes
BI 126 Randy Gallistel: Where Is the Engram?
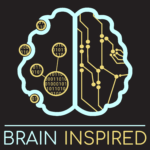
Randy and I discuss his long-standing interest in how the brain stores information to compute. That is, where is the engram, the physical trace of memory in the brain? Modern neuroscience is dominated by the view that memories are stored among synaptic connections in populations of neurons. Randy believes a more reasonable and reliable way to store abstract symbols, like numbers, is to write them into code within individual neurons. Thus, the spiking code, whatever it is, functions to write and read memories into and out of intracellular substrates, like polynucleotides (DNA, RNA, e.g.). He lays out his case in detail in his book with Adam King, Memory and the Computational Brain: Why Cognitive Science will Transform Neuroscience. We also talk about some research and theoretical work since then that support his views.
BI 125 Doris Tsao, Tony Zador, Blake Richards: NAISys
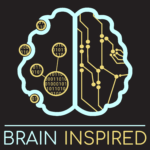
Doris, Tony, and Blake are the organizers for this year’s NAISys conference, From Neuroscience to Artificially Intelligent Systems (NAISys), at Cold Spring Harbor. We discuss the conference itself, some history of the neuroscience and AI interface, their current research interests, and a handful of topics around evolution, innateness, development, learning, and the current and future prospects for using neuroscience to inspire new ideas in artificial intelligence.
BI 124 Peter Robin Hiesinger: The Self-Assembling Brain
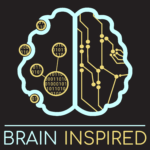
Robin and I discuss many of the ideas in his book The Self-Assembling Brain: How Neural Networks Grow Smarter. The premise is that our DNA encodes an algorithmic growth process that unfolds information via time and energy, resulting in a connected neural network (our brains!) imbued with vast amounts of information from the “start”. This contrasts with modern deep learning networks, which start with minimal initial information in their connectivity, and instead rely almost solely on learning to gain their function. Robin suggests we won’t be able to create anything with close to human-like intelligence unless we build in an algorithmic growth process and an evolutionary selection process to create artificial networks.
BI 123 Irina Rish: Continual Learning
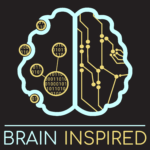
Irina is a faculty member at MILA-Quebec AI Institute and a professor at Université de Montréal. She has worked from both ends of the neuroscience/AI interface, using AI for neuroscience applications, and using neural principles to help improve AI. We discuss her work on biologically-plausible alternatives to back-propagation, using “auxiliary variables” in addition to the normal connection weight updates. We also discuss the world of lifelong learning, which seeks to train networks in an online manner to improve on any tasks as they are introduced. Catastrophic forgetting is an obstacle in modern deep learning, where a network forgets old tasks when it is trained on new tasks. Lifelong learning strategies, like continual learning, transfer learning, and meta-learning seek to overcome catastrophic forgetting, and we talk about some of the inspirations from neuroscience being used to help lifelong learning in networks.
BI 122 Kohitij Kar: Visual Intelligence
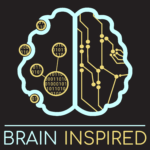
Ko and I discuss a range of topics around his work to understand our visual intelligence. Ko was a postdoc in Jim Dicarlo’s lab, where he helped develop the convolutional neural network models that have become the standard for explaining core object recognition. He is starting his own lab at York University, where he will continue to expand and refine the models, adding important biological details and incorporating models for brain areas outside the ventral visual stream. He will also continue recording neural activity, and performing perturbation studies to better understand the networks involved in our visual cognition.
BI 121 Mac Shine: Systems Neurobiology
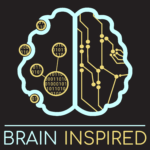
Mac and I discuss his systems level approach to understanding brains, and his theoretical work suggesting important roles for the thalamus, basal ganglia, and cerebellum, shifting the dynamical landscape of brain function within varying behavioral contexts. We also discuss his recent interest in the ascending arousal system and neuromodulators. Mac thinks the neocortex has been the sole focus of too much neuroscience research, and that the subcortical brain regions and circuits have a much larger role underlying our intelligence.
BI 120 James Fitzgerald, Andrew Saxe, Weinan Sun: Optimizing Memories
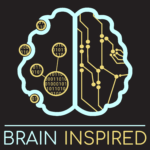
James, Andrew, and Weinan discuss their recent theory about how the brain might use complementary learning systems to optimize our memories. The idea is that our hippocampus creates our episodic memories for individual events, full of particular details. And through a complementary process, slowly consolidates those memories within our neocortex through mechanisms like hippocampal replay. The new idea in their work suggests a way for the consolidated cortical memory to become optimized for generalization, something humans are known to be capable of but deep learning has yet to build. We discuss what their theory predicts about how the “correct” process depends on how much noise and variability there is in the learning environment, how their model solves this, and how it relates to our brain and behavior.
BI 119 Henry Yin: The Crisis in Neuroscience
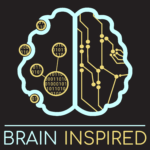
Henry and I discuss why he thinks neuroscience is in a crisis (in the Thomas Kuhn sense of scientific paradigms, crises, and revolutions). Henry thinks our current concept of the brain as an input-output device, with cognition in the middle, is mistaken. He points to the failure of neuroscience to successfully explain behavior despite decades of research. Instead, Henry proposes the brain is one big hierarchical set of control loops, trying to control their output with respect to internally generated reference signals. He was inspired by control theory, but points out that most control theory for biology is flawed by not recognizing that the reference signals are internally generated. Instead, most control theory approaches, and neuroscience research in general, assume the reference signals are what gets externally supplied… by the experimenter.
BI 118 Johannes Jäger: Beyond Networks
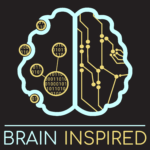
Johannes (Yogi) is a freelance philosopher, researcher & educator. We discuss many of the topics in his online course, Beyond Networks: The Evolution of Living Systems. The course is focused on the role of agency in evolution, but it covers a vast range of topics: process vs. substance metaphysics, causality, mechanistic dynamic explanation, teleology, the important role of development mediating genotypes, phenotypes, and evolution, what makes biological organisms unique, the history of evolutionary theory, scientific perspectivism, and a view toward the necessity of including agency in evolutionary theory. I highly recommend taking his course. We also discuss the role of agency in artificial intelligence, how neuroscience and evolutionary theory are undergoing parallel re-evaluations, and Yogi answers a guest question from Kevin Mitchell.
BI 117 Anil Seth: Being You
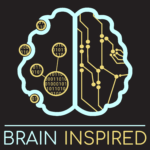
Anil and I discuss a range of topics from his book, BEING YOU A New Science of Consciousness. Anil lays out his framework for explaining consciousness, which is embedded in what he calls the “real problem” of consciousness. You know the “hard problem”, which was David Chalmers term for our eternal difficulties to explain why we have subjective awareness at all instead of being unfeeling, unexperiencing machine-like organisms. Anil’s “real problem” aims to explain, predict, and control the phenomenal properties of consciousness, and his hope is that, by doing so, the hard problem of consciousness will dissolve much like the mystery of explaining life dissolved with lots of good science.
BI 116 Michael W. Cole: Empirical Neural Networks
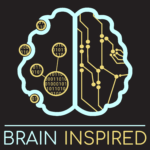
Mike and I discuss his modeling approach to study cognition. Many people I have on the podcast use deep neural networks to study brains, where the idea is to train or optimize the model to perform a task, then compare the model properties with brain properties. Mike’s approach is different in at least two ways. For one, he builds the architecture of his models using structural connectivity data from fMRI recordings. Two, he doesn’t train his models; instead, he uses functional connectivity data from the fMRI recordings to assign weights between nodes of the network (in deep learning, the weights are learned through lots of training). Mike calls his networks empirically-estimated neural networks (ENNs), and/or network coding models. We walk through his approach, what we can learn from models like ENNs, discuss some of his earlier work on cognitive control and our ability to flexibly adapt to new task rules through instruction, and he fields questions from Kanaka Rajan, Kendrick Kay, and Patryk Laurent.
BI 115 Steve Grossberg: Conscious Mind, Resonant Brain
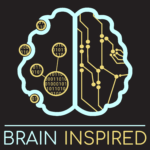
Steve and I discuss his book Conscious Mind, Resonant Brain: How Each Brain Makes a Mind. The book is a huge collection of his models and their predictions and explanations for a wide array of cognitive brain functions. Many of the models spring from his Adaptive Resonance Theory (ART) framework, which explains how networks of neurons deal with changing environments while maintaining self-organization and retaining learned knowledge. ART led Steve to the hypothesis that all conscious states are resonant states, which we discuss. There are also guest questions from György Buzsáki, Jay McClelland, and John Krakauer.
BI 114 Mark Sprevak and Mazviita Chirimuuta: Computation and the Mind
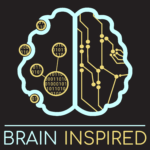
Mark and Mazviita discuss the philosophy and science of mind, and how to think about computations with respect to understanding minds. Current approaches to explaining brain function are dominated by computational models and the computer metaphor for brain and mind. But there are alternative ways to think about the relation between computations and brain function, which we explore in the discussion. We also talk about the role of philosophy broadly and with respect to mind sciences, pluralism and perspectival approaches to truth and understanding, the prospects and desirability of naturalizing representations (accounting for how brain representations relate to the natural world), and much more.
BI 113 David Barack and John Krakauer: Two Views On Cognition
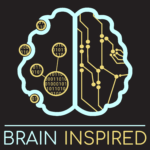
David and John discuss some of the concepts from their recent paper Two Views on the Cognitive Brain, in which they argue the recent population-based dynamical systems approach is a promising route to understanding brain activity underpinning higher cognition. We discuss mental representations, the kinds of dynamical objects being used for explanation, and much more, including David’s perspectives as a practicing neuroscientist and philosopher.
BI ViDA Panel Discussion: Deep RL and Dopamine
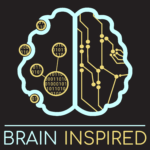
What can artificial intelligence teach us about how the brain uses dopamine to learn? Recent advances in artificial intelligence have yielded novel algorithms for reinforcement
learning (RL), which leverage the power of deep learning together with reward prediction error signals in order to achieve unprecedented performance in complex tasks. In the brain, reward prediction error signals are thought to be signaled by midbrain dopamine neurons and support learning. Can these new advances in Deep RL help us understand the role that dopamine plays in learning? In this panel experts in both theoretical and experimental dopamine research will
discuss this question.
BI 112 Ali Mohebi and Ben Engelhard: The Many Faces of Dopamine
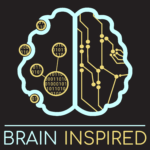
Ali and Ben discuss the ever-expanding discoveries about the roles dopamine plays for our cognition. Dopamine is known to play a role in learning – dopamine (DA) neurons fire when our reward expectations aren’t met, and that signal helps adjust our expectation. Roughly, DA corresponds to a reward prediction error. The reward prediction error has helped reinforcement learning in AI develop into a raging success, specially with deep reinforcement learning models trained to out-perform humans in games like chess and Go. But DA likely contributes a lot more to brain function. We discuss many of those possible roles, how to think about computation with respect to neuromodulators like DA, how different time and spatial scales interact, and more.
BI NMA 06: Advancing Neuro Deep Learning Panel
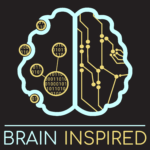
This is the 6th in a series of panel discussions in collaboration with Neuromatch Academy, the online computational neuroscience summer school. This is the 3rd of 3 in the deep learning series. In this episode, the panelists discuss their experiences with advanced topics in deep learning; unsupervised & self-supervised learning, reinforcement learning, continual learning/causality.
BI NMA 05: NLP and Generative Models Panel
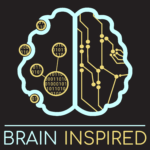
This is the 5th in a series of panel discussions in collaboration with Neuromatch Academy, the online computational neuroscience summer school. This is the 2nd of 3 in the deep learning series. In this episode, the panelists discuss their experiences “doing more with fewer parameters: Convnets, RNNs, attention & transformers, generative models (VAEs & GANs).