All Episodes
BI 159 Chris Summerfield: Natural General Intelligence
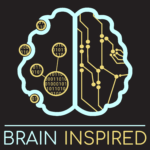
Chris Summerfield runs the Human Information Processing Lab at University of Oxford, and he’s a research scientist at Deepmind. You may remember him from episode 95 with Sam Gershman, when we discussed ideas around the usefulness of neuroscience and psychology for AI. Since then, Chris has released his book, Natural General Intelligence: How understanding the brain can help us build AI. In the book, Chris makes the case that inspiration and communication between the cognitive sciences and AI is hindered by the different languages each field speaks. But in reality, there has always been and still is a lot of overlap and convergence about ideas of computation and intelligence, and he illustrates this using tons of historical and modern examples.
BI 158 Paul Rosenbloom: Cognitive Architectures
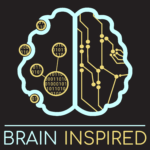
Paul Rosenbloom is Professor Emeritus of Computer Science at the University of Southern California. In the early 1980s, Paul , along with John Laird and the early AI pioneer Alan Newell, developed one the earliest and best know cognitive architectures called SOAR. A cognitive architecture, as Paul defines it, is a model of the fixed structures and processes underlying minds, and in Paul’s case the human mind. And SOAR was aimed at generating general intelligence. He doesn’t work on SOAR any more, although SOAR is still alive and well in the hands of his old partner John Laird. He did go on to develop another cognitive architecture, called Sigma, and in the intervening years between those projects, among other things Paul stepped back and explored how our various scientific domains are related, and how computing itself should be considered a great scientific domain. That’s in his book On Computing: The Fourth Great Scientific Domain.
BI 157 Sarah Robins: Philosophy of Memory
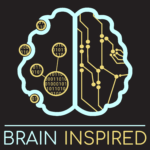
Sarah Robins is a philosopher at the University of Kansas, one a growing handful of philosophers specializing in memory. Much of her work focuses on memory traces, which is roughly the idea that somehow our memories leave a trace in our minds. We discuss memory traces themselves and how they relate to the engram (see BI 126 Randy Gallistel: Where Is the Engram?, and BI 127 Tomás Ryan: Memory, Instinct, and Forgetting).
BI 156 Mariam Aly: Memory, Attention, and Perception
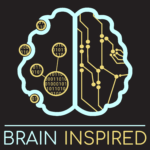
Mariam Aly runs the Aly lab at Columbia University, where she studies the interaction of memory, attention, and perception in brain regions like the hippocampus. The short story is that memory affects our perceptions, attention affects our memories, memories affect our attention, and these effects have signatures in neural activity measurements in our hippocampus and other brain areas. We discuss her experiments testing the nature of those interactions. We also discuss a particularly difficult stretch in Mariam’s graduate school years, and how she now prioritizes her mental health.
BI 155 Luiz Pessoa: The Entangled Brain
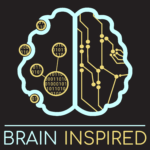
Luiz Pessoa runs his Laboratory of Cognition and Emotion at the University of Maryland, College Park, where he studies how emotion and cognition interact. On this episode, we discuss many of the topics from his latest book,
The Entangled Brain: How Perception, Cognition, and Emotion Are Woven Together, which is aimed at a general audience. The book argues we need to re-think how to study the brain.
BI 154 Anne Collins: Learning with Working Memory
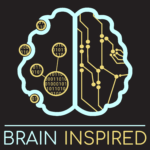
Anne Collins runs her Computational Cognitive Neuroscience Lab at the University of California, Berkley One of the things she’s been working on for years is how our working memory plays a role in learning as well, and specifically how working memory and reinforcement learning interact to affect how we learn, depending on the nature of what we’re trying to learn. We discuss that interaction specifically. We also discuss more broadly how segregated and how overlapping and interacting our cognitive functions are, what that implies about our natural tendency to think in dichotomies – like MF vs MB-RL, system-1 vs system-2, etc., and we dive into plenty other subjects, like how to possibly incorporate these ideas into AI.
BI 153 Carolyn Dicey-Jennings: Attention and the Self
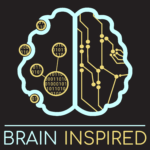
Carolyn Dicey Jennings is a philosopher and a cognitive scientist at University of California, Merced. In her book The Attending Mind, she lays out an attempt to unify the concept of attention. Carolyn defines attention roughly as the mental prioritization of some stuff over other stuff based on our collective interests. And one of her main claims is that attention is evidence of a real, emergent self or subject, that can’t be reduced to microscopic brain activity. She does connect attention to more macroscopic brain activity, suggesting slow longer-range oscillations in our brains can alter or entrain the activity of more local neural activity, and this is a candidate for mental causation. We unpack that more in our discussion, and how Carolyn situates attention among other cognitive functions, like consciousness, action, and perception.
BI 152 Michael L. Anderson: After Phrenology: Neural Reuse
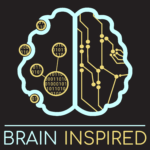
Michael L. Anderson is a professor at the Rotman Institute of Philosophy, at Western University. His book, After Phrenology: Neural Reuse and the Interactive Brain, calls for a re-conceptualization of how we understand and study brains and minds. Neural reuse is the phenomenon that any given brain area is active for multiple cognitive functions, and partners with different sets of brain areas to carry out different cognitive functions. We discuss the implications for this, and other topics in Michael’s research and the book, like evolution, embodied cognition, and Gibsonian perception. Michael also fields guest questions from John Krakauer and Alex Gomez-Marin, about representations and metaphysics, respectively.
BI 151 Steve Byrnes: Brain-like AGI Safety
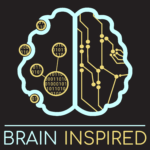
Steve Byrnes is a physicist turned AGI safety researcher. He’s concerned that when we create AGI, whenever and however that might happen, we run the risk of creating it in a less than perfectly safe way. AGI safety (AGI not doing something bad) is a wide net that encompasses AGI alignment (AGI doing what we want it to do). We discuss a host of ideas Steve writes about in his Intro to Brain-Like-AGI Safety blog series, which uses what he has learned about brains to address how we might safely make AGI.
BI 150 Dan Nicholson: Machines, Organisms, Processes
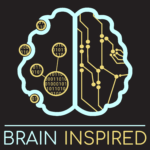
Dan Nicholson is a philosopher at George Mason University. He incorporates the history of science and philosophy into modern analyses of our conceptions of processes related to life and organisms. He is also interested in re-orienting our conception of the universe as made fundamentally of things/substances, and replacing it with the idea the universe is made fundamentally of processes (process philosophy). In this episode, we both of those subjects, the why the “machine conception of the organism” is incorrect, how to apply these ideas to topics like neuroscience and artificial intelligence, and much more.
BI 149 William B. Miller: Cell Intelligence
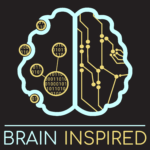
William B. Miller is an ex-physician turned evolutionary biologist. In this episode, we discuss topics related to his new book, Bioverse: How the Cellular World Contains the Secrets to Life’s Biggest Questions. The premise of the book is that all individual cells are intelligent in their own right, and possess a sense of self. From this, Bill makes the case that cells cooperate with other cells to engineer whole organisms that in turn serve as wonderful hosts for the myriad cell types. Further, our bodies are collections of our own cells (with our DNA), and an enormous amount and diversity of foreign cells – our microbiome – that communicate and cooperate with each other and with our own cells. We also discuss how cell intelligence compares to human intelligence, what Bill calls the “era of the cell” in science, how the future of medicine will harness the intelligence of cells and their cooperative nature, and much more.
BI 148 Gaute Einevoll: Brain Simulations
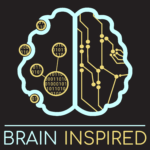
Gaute Einevoll is a professor at the University of Oslo and Norwegian University of Life Sciences. Use develops detailed models of brain networks to use as simulations, so neuroscientists can test their various theories and hypotheses about how networks implement various functions. Thus, the models are tools. The goal is to create models that are multi-level, to test questions at various levels of biological detail; and multi-modal, to predict that handful of signals neuroscientists measure from real brains (something Gaute calls “measurement physics”). We also discuss Gaute’s thoughts on Carina Curto’s “beautiful vs ugly models”, and his reaction to Noah Hutton’s In Silico documentary about the Blue Brain and Human Brain projects (Gaute has been funded by the Human Brain Project since its inception).
BI 147 Noah Hutton: In Silico
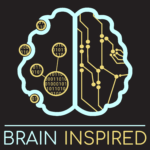
Noah Hutton writes, directs, and scores documentary and narrative films. On this episode, we discuss his documentary In Silico. In 2009, Noah watched a TED talk by Henry Markram, in which Henry claimed it would take 10 years to fully simulate a human brain. This claim inspired Noah to chronicle the project, visiting Henry and his team periodically throughout. The result was In Silico, which tells the science, human, and social story of Henry’s massively funded projects – the Blue Brain Project and the Human Brain Project.
BI 146 Lauren Ross: Causal and Non-Causal Explanation
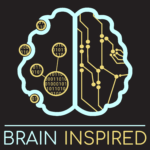
Lauren Ross is an Associate Professor at the University of California, Irvine. She studies and writes about causal and non-causal explanations in philosophy of science, including distinctions among causal structures. Throughout her work, Lauren employs Jame’s Woodward’s interventionist approach to causation, which Jim and I discussed in episode 145. In this episode, we discuss Jim’s lasting impact on the philosophy of causation, the current dominance of mechanistic explanation and its relation to causation, and various causal structures of explanation, including pathways, cascades, topology, and constraints.
BI 145 James Woodward: Causation with a Human Face
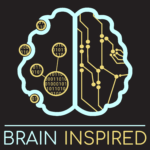
James Woodward is a recently retired Professor from the Department of History and Philosophy of Science at the University of Pittsburgh. Jim has tremendously influenced the field of causal explanation in the philosophy of science. His account of causation centers around intervention – intervening on a cause should alter its effect. From this minimal notion, Jim has described many facets and varieties of causal structures. In this episode, we discuss topics from his recent book, Causation with a Human Face: Normative Theory and Descriptive Psychology. In the book, Jim advocates that how we should think about causality – the normative – needs to be studied together with how we actually do think about causal relations in the world – the descriptive. We discuss many topics around this central notion, epistemology versus metaphysics, the the nature and varieties of causal structures.
BI 144 Emily M. Bender and Ev Fedorenko: Large Language Models
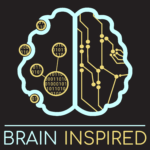
Large language models, often now called “foundation models”, are the model de jour in AI, based on the transformer architecture. In this episode, I bring together Evelina Fedorenko and Emily M. Bender to discuss how language models stack up to our own language processing and generation (models and brains both excel at next-word prediction), whether language evolved in humans for complex thoughts or for communication (communication, says Ev), whether language models grasp the meaning of the text they produce (Emily says no), and much more.
BI 143 Rodolphe Sepulchre: Mixed Feedback Control
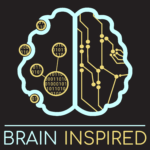
Rodolphe Sepulchre is a control engineer and theorist at Cambridge University. He focuses on applying feedback control engineering principles to build circuits that model neurons and neuronal circuits. We discuss his work on mixed feedback control – positive and negative – as an underlying principle of the mixed digital and analog brain signals,, the role of neuromodulation as a controller, applying these principles to Eve Marder’s lobster/crab neural circuits, building mixed-feedback neuromorphics, some feedback control history, and how “If you wish to contribute original work, be prepared to face loneliness,” among other topics
BI 142 Cameron Buckner: The New DoGMA
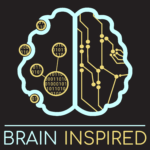
Cameron Buckner is a philosopher and cognitive scientist at The University of Houston. He is writing a book about the age-old philosophical debate on how much of our knowledge is innate (nature, rationalism) versus how much is learned (nurture, empiricism). In the book and his other works, Cameron argues that modern AI can help settle the debate. In particular, he suggests we focus on what types of psychological “domain-general faculties” underlie our own intelligence, and how different kinds of deep learning models are revealing how those faculties may be implemented in our brains. The hope is that by building systems that possess the right handful of faculties, and putting those systems together in a way they can cooperate in a general and flexible manner, it will result in cognitive architectures we would call intelligent. Thus, what Cameron calls The New DoGMA: Domain-General Modular Architecture. We also discuss his work on mental representation and how representations get their content – how our thoughts connect to the natural external world.